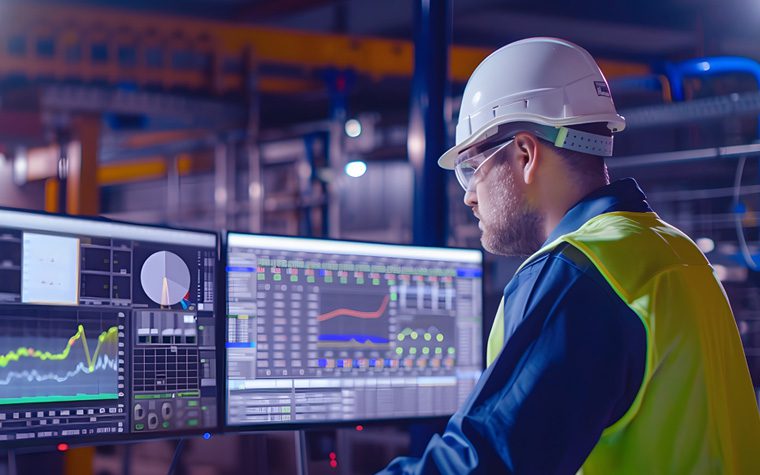
Implementing Predictive Maintenance in Plant Operations
Predictive maintenance (PdM) has become a cornerstone of modern plant operations, providing a strategic approach to equipment maintenance that minimizes downtime, enhances efficiency, and reduces costs. By leveraging advanced technologies such as the Internet of Things (IoT), machine learning, and data analytics, predictive maintenance allows plant operators to anticipate equipment failures before they occur.
This proactive strategy ensures that maintenance activities are performed only when necessary, thereby extending the life of equipment and optimizing plant performance.
In this blog, let’s explore the key aspects of implementing predictive maintenance in plant operations, including its benefits, the technologies involved, and the steps to successfully deploy a predictive maintenance program.
Understanding Predictive Maintenance
Predictive maintenance is a data-driven approach that monitors the condition of equipment in real-time to predict when maintenance should be performed. Unlike reactive maintenance, which addresses equipment failures after they occur, or preventive maintenance, which schedules maintenance at regular intervals regardless of equipment condition, predictive maintenance focuses on performing maintenance only when data indicates that a failure is imminent.
Benefits of Predictive Maintenance
- Reduced Downtime: By predicting equipment failures before they happen, predictive maintenance minimizes unexpected downtime, ensuring continuous plant operations.
- Cost Savings: Avoiding unnecessary maintenance and preventing catastrophic equipment failures can significantly reduce maintenance costs.
- Extended Equipment Life: Regular monitoring and timely maintenance can extend the lifespan of equipment.
- Improved Safety: Predictive maintenance helps identify potential safety hazards before they become critical, enhancing workplace safety.
- Optimal Resource Utilization: Maintenance resources are used more efficiently, as interventions are based on actual equipment condition rather than arbitrary schedules.
Key Technologies in Predictive Maintenance
Implementing predictive maintenance involves several advanced technologies:
- Internet of Things (IoT): IoT devices, such as sensors and actuators, are installed on equipment to collect real-time data on parameters like temperature, vibration, pressure, and humidity.
- Data Analytics: Advanced data analytics tools process and analyze the collected data to identify patterns and predict equipment failures.
- Machine Learning: Machine learning algorithms learn from historical data to improve the accuracy of failure predictions over time.
- Cloud Computing: Cloud platforms store and process large volumes of data, providing scalability and flexibility for predictive maintenance systems.
- Digital Twins: Digital twins are virtual replicas of physical assets that simulate their behavior and predict potential failures based on real-time data.
Steps to Implement Predictive Maintenance
- Assess Current Maintenance Practices
The first step in implementing predictive maintenance is to evaluate existing maintenance practices. This involves analyzing the current maintenance strategy, identifying pain points, and determining the potential benefits of predictive maintenance for the plant.
- Identify Critical Equipment
Not all equipment requires predictive maintenance. Focus on critical equipment that has a significant impact on plant operations, safety, or costs. Prioritize assets that are prone to frequent failures or have high maintenance costs.
- Install IoT Sensors
Install IoT sensors on the identified critical equipment to collect real-time data. The type and number of sensors depend on the equipment and the parameters to be monitored. Ensure that the sensors are properly calibrated and maintained to provide accurate data.
- Collect and Store Data
Implement a data collection system to gather and store data from the sensors. This system should be capable of handling large volumes of data and integrating with existing plant systems. Cloud platforms are often used for this purpose due to their scalability and flexibility.
- Analyze Data
Use data analytics tools to process and analyze the collected data. These tools should be able to identify patterns, detect anomalies, and predict potential failures. Machine learning algorithms can be trained on historical data to improve prediction accuracy.
- Develop Predictive Models
Develop predictive models based on the analyzed data. These models should be able to predict equipment failures with a high degree of accuracy. Validate the models using historical data and refine them as needed.
- Integrate with Maintenance Systems
Integrate the predictive maintenance system with the plant’s existing maintenance management system. This allows maintenance activities to be scheduled automatically based on the predictions, ensuring timely interventions.
- Implement and Monitor
Implement the predictive maintenance system and continuously monitor its performance. Regularly review the predictions and maintenance activities to ensure that the system is functioning as expected. Make adjustments as needed to improve accuracy and effectiveness.
Challenges in Implementing Predictive Maintenance
Implementing predictive maintenance is not without its challenges. Some of the common obstacles include:
- High Initial Costs: The cost of installing sensors, setting up data infrastructure, and developing predictive models can be high.
- Data Management: Handling large volumes of data and ensuring its accuracy can be challenging.
- Skill Requirements: Predictive maintenance requires expertise in data analytics, machine learning, and IoT, which may not be readily available within the organization.
- Integration with Existing Systems: Integrating predictive maintenance systems with existing plant systems can be complex and time-consuming.
- Change Management: Shifting from traditional maintenance practices to predictive maintenance requires a cultural change within the organization, which can be difficult to achieve.
Overcoming the Challenges
To overcome these challenges, consider the following strategies:
- Start Small: Begin with a pilot project focusing on a few critical assets. This allows you to test the system and make adjustments before scaling up.
- Leverage Existing Infrastructure: Where possible, use existing data infrastructure and integrate predictive maintenance with current systems to reduce costs and complexity.
- Invest in Training: Provide training for staff to develop the necessary skills in data analytics, machine learning, and IoT.
- Work with Experts: Partner with experts or vendors who specialize in predictive maintenance to ensure a smooth implementation process.
- Communicate Benefits: Clearly communicate the benefits of predictive maintenance to all stakeholders to gain buy-in and support for the initiative.
Predictive maintenance offers a powerful approach to optimizing plant operations by preventing unexpected equipment failures, reducing maintenance costs, and enhancing overall efficiency. By leveraging advanced technologies such as IoT, data analytics, and machine learning, plant operators can transition from reactive to proactive maintenance strategies.
While implementing predictive maintenance can be challenging, starting with a pilot project, leveraging existing infrastructure, investing in training, and working with experts can help overcome these obstacles. As technology continues to advance, predictive maintenance will become an increasingly essential component of modern plant operations, driving continuous improvement and operational excellence.